WASHINGTON: The military is investing heavily in artificial intelligence — but AI alone is not enough. You need hardware that can handle your high-powered algorithms, without taking up so much space, weight, and power that it can’t fit into a frontline command post, fighter jet, or tank. And that’s not easy.
There’s “a changing demand signal” from the Defense Department, said Bill Conley, the incoming CTO of electronics maker Mercury Systems. He should know: He just left government after a career that took him from a Navy lab to DARPA to the Office of the Secretary of Defense, where he was director for electronic warfare.
“Over the last couple of years there’s been a substantial amount of effort dedicated [to] the next generation of artificial intelligence, algorithm training, data,” Conley told me in an interview, “[but] at the tactical edge, not only do you need the algorithms and the data… you also need the hardware it actually runs on.”
In Silicon Valley and academia, that generally means a cluster or cloud of computers all working together. That’s not something you can throw in the back of your Humvee or install in an aircraft’s cockpit.
Yes, the military’s controversial JEDI program is trying to bring cloud computing to frontline units, so they can remotely access powerful server farms. But the Pentagon doesn’t expect troops to have continuous access to the cloud in the face of enemy hacking and jamming.
Now, for some functions, intermittent access is okay, if not ideal. Consider military intelligence. The big AI brain can reside on the remote servers, with troops uploading their reports and downloading the AI’s analysis whenever they get a working connection. But if you need something to work all the time — say, an electronic warfare system to jam incoming missiles’ guidance systems before they kill you — you can’t rely on a distant cloud. You need to bring your AI with you. But how?
For a half century, DRS has provided military forces around the world with advanced technologies and capabilities to meet their mission needs. Here are some highlights.
From LEONARDO DRS
“You’re not going to set up some giant cloud server in Syria at a forward operating base,” agreed Paul Scharre, an Army Ranger turned defense analyst at the Center for a New American Security. But as technology improves, everything gets smaller.
Just how small you need to get your hardware depends a lot on two factors, Scharre said.
The most obvious issue is the size of the platform you’re trying to fit onto. “Whether you’re talking about a Humvee or a fighter jet or an aircraft carrier makes a big difference,” he said.
An equally important but less intuitive factor is the size of your AI application. If you want to actively train machine-learning algorithms, that requires tremendous computing power and data storage. But once the training’s complete, the finished algorithm requires much less power to run — frequently less than a tenth of a percent as much.
“What’s really compute-intensive is really the big data-driven deep learning,” Scharre told me. “You’re going to have to do that at a cloud center, that’s not going to happen at the edge.”
In fact, “you probably don’t want your systems to be learning autonomously at the edge,” Scharre argued, because the algorithms might evolve in unpredictable or even counterproductive ways.
That’s true for many missions, Conley countered — but not for all. There are important AI applications that require the machine learning algorithms to keep learning continuously, in the field, as the situation they’re coping with changes. That’s particularly important for what’s called cognitive electronic warfare: systems that can detect a never-before-encountered transmission that’s not in any pre-loaded data library, analyze it on the spot, decide whether its source is hostile or not, and devise a counter-signal to jam it if necessary.
“I expect a future battlespace will contain threat signals not previously observed, [so] it will be essential for many platforms to be executing realtime decision algorithms,” Conley said, “[and] reinforcement learning, particularly real-time decision algorithms cannot be fully trained during the development process.” You have to be able to run them in the real world.
That means you need a lot of computing power on relatively compact hardware that doesn’t require too much space, weight, electrical power, or cooling. (Cooling is a huge issue for computers: Even a laptop or a smartphone will be hot to the touch if it runs hard and long enough, and chips don’t work when they overheat).
The chance to work on this “next generation of hardware” is a major reason Conley took the job at Mercury, whose core business is building sensors and processors. An engineer by training, he started his government career in the lab before getting promoted to more managerial and policy-oriented positions. Now, he said, he’s getting back to technology, “which I’m really excited about.”
The next-gen hardware has another major advantage, Conley continued. Not only is it more compact and less ravenous for electricity, it’s also potentially much more secure against sabotage in the supply chain, which is a major worry for the Defense Department.
There’s been tremendous progress in fitting ever larger numbers of ever tinier components on a computer chip, Scharre said, which is what drives the exponential improvement known as Moore’s law. (That said, he warned, we’ll soon hit fundamental limits of scale on the atomic level). But the price of that progress is that it requires ever more sophisticated and expensive equipment to build the nano-miniaturized components.
It now costs $10 billion to $20 billion to stand up a new chip fabrication factory, Scharre told me. Those huge costs drive ever greater centralization and consolidation, most of it overseas — especially in Taiwan, which is increasingly economically intertwined with mainland China.
So Scharre doesn’t worry so much about industry’s ability to build the desired hardware — he’s worried about whether we can trust it not to be riddled with bugs or deliberate backdoors. “I’m more concerned abut the ability of DoD actually to access secure cutting edge hardware that is trustworthy,” he said, “that we know hasn’t’ been exploited by some adversary in a foreign production facility.”
In most cases the military can’t afford to subsidize a dedicated production infrastructure on US soil. Even in cases where there are so-called trusted foundries run by US citizens, Conley noted, there’s still a potential insider threat — the possibility that one of those citizens could be suborned.
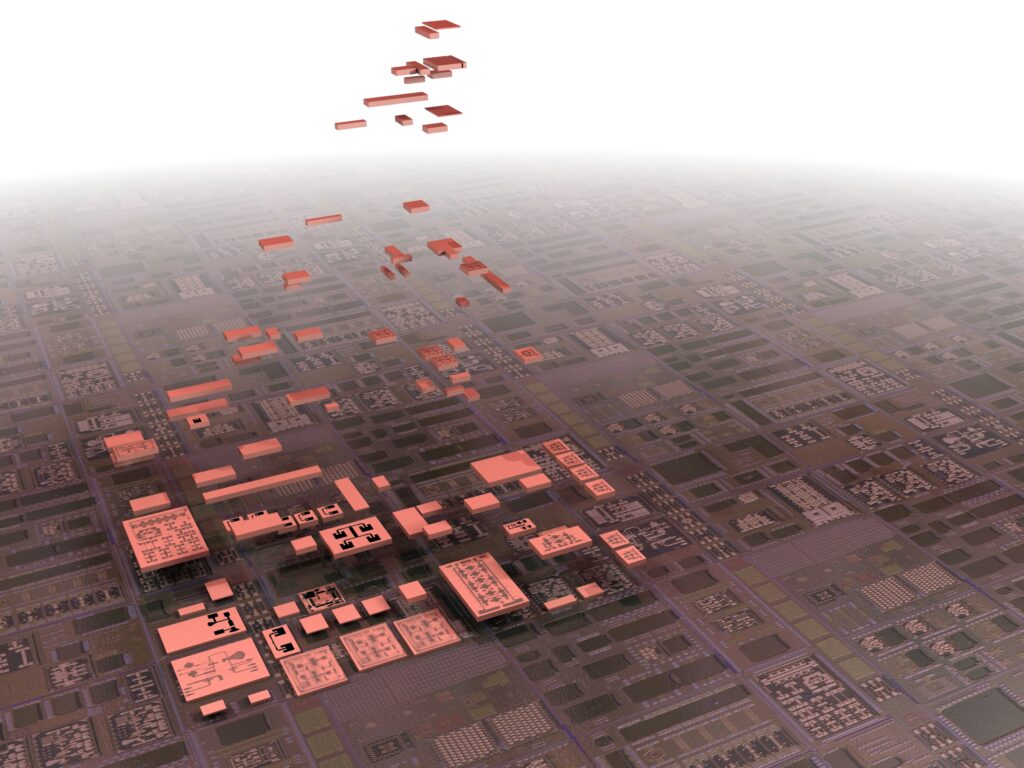
DARPA concept for advanced microchip with modular subcomponents.
A Six Fingered Glove
But there’s a hardware solution here too, Conely said. But you don’t have to trust the humans: You can use the different subcomponents on the chip itself to check each other. The latest microelectronics are so complex, Conley said, with so many different interdependent parts, that a saboteur can’t meddle with one of them without affecting the rest — and you can design your diagnostics to detect that. Subvert even a single subcomponent of the chip, he said, and it’ll function differently enough that it won’t pass system-level testing.
The difference is as obvious, he said, as a six-fingered glove. As soon as you put it on your hand, you know something is wrong.
With this approach, since you can catch bad subcomponents in testing, you don’t have to spend as much effort ensuring your suppliers are 100 percent reliable. That allows you to go to regular commercial vendors and get much lower prices — plus, often, much more up-to-date technology than available in the defense industrial base.
Mercury itself serves both defense and commercial customers, Conley said, but it is managed like a commercial company. That includes investing much more of its revenue in independent (non-government-funded) R&D — 11 to 15 percent — than the typical defense contractor, he said. And that, he argued, is the kind of investment it’ll take to deliver the next-generation hardware required to run artificial intelligence on the battlefield.